Продолжая использовать сайт, вы даете свое согласие на работу с этими файлами.
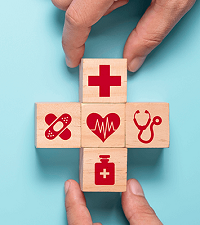
LocDB
![]() | |
Content | |
---|---|
Description | experimental annotations of localization |
Organisms |
Homo sapiens Arabidopsis thaliana |
Contact | |
Research center | Columbia University |
Laboratory | Department of Biochemistry and Molecular Biophysics |
Authors | Shruti Rastogi |
Primary citation | Rastogi & al. (2011) |
Release date | 2010 |
Access | |
Data format | MySQL database |
Website | http://www.rostlab.org/services/locDB |
Web service URL | http://www.rostlab.org/services/locDB/search.php |
Miscellaneous | |
Version | Release 1.0 |
LocDB is an expert-curated database that collects experimental annotations for the subcellular localization of proteins in Homo sapiens (human) and Arabidopsis thaliana (Weed). The database also contains predictions of subcellular localization from a variety of state-of-the-art prediction methods for all proteins with experimental information.
Proteins are the fundamental functional components of cells. They are responsible for transforming genetic information into physical reality. These macromolecules mediate gene regulation, enzymatic catalysis, cellular metabolism, DNA replication, and transport of nutrients, recognition, and transmission of signals. The interpretation of this wealth of data to elucidate protein function in post-genomic era is a fundamental challenge. To date, even for the most well-studied organisms such as yeast, about one-fourth of the proteins remain uncharacterized. A major obstacle in experimentally determining protein function is that the studies require enormous resources. Hence, the gap between the amount of sequences deposited in databases and the experimental characterization of the corresponding proteins is ever-growing. Bioinformatics plays a central role in bridging this sequence-function gap through the development of tools for faster and more effective prediction of protein function. This repository effectively fills the gap between experimental annotations and predictions and provides a bigger and more reliable dataset for the testing of new prediction methods.