Продолжая использовать сайт, вы даете свое согласие на работу с этими файлами.
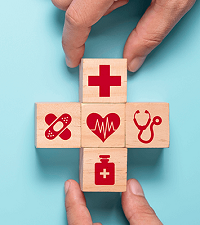
Basic reproduction number
In epidemiology, the basic reproduction number, or basic reproductive number (sometimes called basic reproduction ratio or basic reproductive rate), denoted (pronounced R nought or R zero), of an infection is the expected number of cases directly generated by one case in a population where all individuals are susceptible to infection. The definition assumes that no other individuals are infected or immunized (naturally or through vaccination). Some definitions, such as that of the Australian Department of Health, add the absence of "any deliberate intervention in disease transmission". The basic reproduction number is not necessarily the same as the effective reproduction number
(usually written
[t for time], sometimes
), which is the number of cases generated in the current state of a population, which does not have to be the uninfected state.
is a dimensionless number (persons infected per person infecting) and not a time rate, which would have units of time−1, or units of time like doubling time.
is not a biological constant for a pathogen as it is also affected by other factors such as environmental conditions and the behaviour of the infected population.
values are usually estimated from mathematical models, and the estimated values are dependent on the model used and values of other parameters. Thus values given in the literature only make sense in the given context and it is recommended not to use obsolete values or compare values based on different models.
does not by itself give an estimate of how fast an infection spreads in the population.
The most important uses of are determining if an emerging infectious disease can spread in a population and determining what proportion of the population should be immunized through vaccination to eradicate a disease. In commonly used infection models, when
the infection will be able to start spreading in a population, but not if
. Generally, the larger the value of
, the harder it is to control the epidemic. For simple models, the proportion of the population that needs to be effectively immunized (meaning not susceptible to infection) to prevent sustained spread of the infection has to be larger than
. This is the so-called Herd immunity threshold or herd immunity level. Here, herd immunity means that the disease cannot spread in the population because each infected person, on average, can only transmit the infection to less than one other contact. Conversely, the proportion of the population that remains susceptible to infection in the endemic equilibrium is
. However, this threshold is based on simple models that assume a fully mixed population with no structured relations between the individuals. For example, if there is some correlation between people's immunization (e.g., vaccination) status, then the formula
may underestimate the herd immunity threshold.
The basic reproduction number is affected by several factors, including the duration of infectivity of affected people, the infectiousness of the microorganism, and the number of susceptible people in the population that the infected people contact.
History
The roots of the basic reproduction concept can be traced through the work of Ronald Ross, Alfred Lotka and others, but its first modern application in epidemiology was by George Macdonald in 1952, who constructed population models of the spread of malaria. In his work he called the quantity basic reproduction rate and denoted it by . "Rate" in this context means per person, which makes
dimensionless as required. Because this can be misleading to anyone who understands "rate" only in the sense per unit of time, "number" or "ratio" is now preferred.
Definitions in specific cases
Contact rate and infectious period
Suppose that infectious individuals make an average of infection-producing contacts per unit time, with a mean infectious period of
. Then the basic reproduction number is:
where is the rate of contact between susceptible and infected individuals and
is the transmissibility, i.e, the probability of infection given a contact. It is also possible to decrease the infectious period
by finding and then isolating, treating or eliminating (as is often the case with animals) infectious individuals as soon as possible.
With varying latent periods
Latent period is the transition time between contagion event and disease manifestation. In cases of diseases with varying latent periods, the basic reproduction number can be calculated as the sum of the reproduction numbers for each transition time into the disease. An example of this is tuberculosis (TB). Blower and coauthors calculated from a simple model of TB the following reproduction number:
Heterogeneous populations
In populations that are not homogeneous, the definition of is more subtle. The definition must account for the fact that a typical infected individual may not be an average individual. As an extreme example, consider a population in which a small portion of the individuals mix fully with one another while the remaining individuals are all isolated. A disease may be able to spread in the fully mixed portion even though a randomly selected individual would lead to fewer than one secondary case. This is because the typical infected individual is in the fully mixed portion and thus is able to successfully cause infections. In general, if the individuals infected early in an epidemic are on average either more likely or less likely to transmit the infection than individuals infected late in the epidemic, then the computation of
must account for this difference. An appropriate definition for
in this case is "the expected number of secondary cases produced, in a completely susceptible population, produced by a typical infected individual".
The basic reproduction number can be computed as a ratio of known rates over time: if an infectious individual contacts other people per unit time, if all of those people are assumed to contract the disease, and if the disease has a mean infectious period of
, then the basic reproduction number is just
. Some diseases have multiple possible latency periods, in which case the reproduction number for the disease overall is the sum of the reproduction number for each transition time into the disease.
Epidemic Models on Networks
In reality, diseases spread over networks of contact between people. Such a network can be represented mathematically with a graph and is called the contact network. Every node in a contact network is a representation of an individual and each link (edge) between a pair of nodes represents the contact between them. Links in the contact networks may be used to transmit the disease between the individuals and each disease has its own dynamics on top of its contact network. For example, individuals in a population can be assigned to compartments with labels – for example, S, I, or R, (Susceptible, Infectious, or Recovered) and they progress between compartments. The order of the labels usually shows the flow patterns between the compartments; for instance, SIR means each individual is originally susceptible then changes to infectious and finally gets recovered and remained recovered (immune) forever. On the other hand, public health may apply some interventions such as vaccination or contact tracing to reduce the spread of an epidemic disease. The combination of disease dynamics under the influence of interventions, if any, on a contact network may be modeled with another network, known as a transmission network. In a transmission network, all the links are responsible for transmitting the disease. If such a network is a locally tree-like network, meaning that any local neighborhood in such a network takes the form of a tree, then the basic reproduction can be written in terms of the average excess degree of the transmission network such that:
where is the mean-degree (average degree) of the network and
is the second moment of the transmission network degree distribution. It is, however, not always straightforward to find the transmission network out of the contact network and the disease dynamics. For example, if a contact network can be approximated with an Erdős–Rényi graph with a Poissonian degree distribution, and the disease spreading parameters are as defined in the example above, such that
is the transmission rate per person and the disease has a mean infectious period of
, then the basic reproduction number is
since
for a Poisson distribution.
Compartmental models in epidemiology
Next-generation method
One way to calculate is to average the expected number of new infections over all possible infected types. The next-generation method is a general method of deriving
when more than one class of infectives is involved. This method, originally introduced by Diekmann et al. (1990), can be used for models with underlying age structure or spatial structure, among other possibilities. In this picture, the spectral radius of the next-generation matrix
gives the basic reproduction number,
Consider a sexually transmitted disease. In a naive population where almost everyone is susceptible, but the infection seed, if the expected number of gender 1 is and the expected number of infected gender 2 is
, we can know how many would be infected in the next-generation. Such that the next-generation matrix
can be written as:
The spectral radius of the next-generation matrix is the basic reproduction number, , that is here, the geometric mean of the expected number of each gender in the next-generation. Note that multiplication factors
and
alternate because, the infectious person has to ‘pass through’ a second gender before it can enter a new host of the first gender. In other words, it takes two generations to get back to the same type, and every two generations numbers are multiplied by
×
. The average per generation multiplication factor is therefore
. Note that
is a non-negative matrix so it has single, unique, positive, real eigenvalue which is strictly greater than all the others.
Next-generation matrix for compartmental models
In mathematical modelling of infectious disease, the dynamics of spreading is usually described through a set of non-linear ordinary differential equations (ODE). So there is always coupled equations of form
which shows how the number of people in compartment
changes over time. For example, in a SIR model,
,
, and
. Compartmental models have a disease-free equilibrium (DFE) meaning that it is possible to find an equilibrium while setting the number of infected people to zero,
. In other words, as a rule, there is an infection-free steady state. This solution, also usually ensures that the disease-free equilibrium is also an equilibrium of the system. There is another fixed point known as an Endemic Equilibrium (EE) where the disease is not totally eradicated and remains in the population. Mathematically,
is a threshold for stability of a disease-free equilibrium such that:
To calculate , the first step is to linearise around the disease-free equilibrium (DFE), but for the infected subsystem of non-linear ODEs which describe the production of new infections and changes in state among infected individuals. Epidemiologically, the linearisation reflects that
characterizes the potential for initial spread of an infectious person in a naive population, assuming the change in the susceptible population is negligible during the initial spread. A linear system of ODEs can always be described by a matrix. So, the next step is to construct a linear positive operator that provides the next generation of infected people when applied to the present generation. Note that this operator (matrix) is responsible for the number of infected people, not all the compartments. Iteration of this operator describes the initial progression of infection within the heterogeneous population. So comparing the spectral radius of this operator to unity determines whether the generations of infected people grow or not.
can be written as a product of the infection rate near the disease-free equilibrium and average duration of infectiousness. It is used to find the peak and final size of an epidemic.
The SEIR model with vital dynamics and constant population
As described in the example above, so many epidemic processes can be described with a SIR model. However, for many important infections, such as COVID-19, there is a significant latency period during which individuals have been infected but are not yet infectious themselves. During this period the individual is in compartment E (for exposed). Here, the formation of the next-generation matrix from the SEIR model involves determining two compartments, infected and non-infected, since they are the populations that spread the infection. So we only need to model the exposed, E, and infected, I, compartments. Consider a population characterized by a death rate and birth rate
where a communicable disease is spreading. As in the previous example, we can use the transition rates between the compartments per capita such that
be the infection rate,
be the recovery rate, and
be the rate at which a latent individual becomes infectious. Then, we can define the model dynamics using the following equations:
We can now make matrices of partial derivatives of and
such that
and
, where
is the disease-free equilibrium.
We now can form the next-generation matrix (operator) . Basically,
is a non-negative matrix which represents the infection rates near the equilibrium, and
is an M-matrix for linear transition terms making
a matrix which represents the average duration of infectiousness. Therefore,
gives the rate at which infected individuals in
produce new infections in
, times the average length of time an individual spends in a single visit to compartment
Finally, for this SEIR process we can have:
and
and so
Estimation methods
The basic reproduction number can be estimated through examining detailed transmission chains or through genomic sequencing. However, it is most frequently calculated using epidemiological models. During an epidemic, typically the number of diagnosed infections over time
is known. In the early stages of an epidemic, growth is exponential, with a logarithmic growth rate
In exponential growth, is related to the doubling time
as
Simple model
If an individual, after getting infected, infects exactly new individuals only after exactly a time
(the serial interval) has passed, then the number of infectious individuals over time grows as
For example, with and
, we would find
.
If is time dependent
Latent infectious period, isolation after diagnosis
In this model, an individual infection has the following stages:
- Exposed: an individual is infected, but has no symptoms and does not yet infect others. The average duration of the exposed state is
.
- Latent infectious: an individual is infected, has no symptoms, but does infect others. The average duration of the latent infectious state is
. The individual infects
other individuals during this period.
- Isolation after diagnosis: measures are taken to prevent further infections, for example by isolating the infected person.
This is a SEIR model and may be written in the following form
In the special case , this model results in
, which is different from the simple model above (
). For example, with the same values
and
, we would find
, rather than the true value of
. The difference is due to a subtle difference in the underlying growth model; the matrix equation above assumes that newly infected patients are currently already contributing to infections, while in fact infections only occur due to the number infected at
ago. A more correct treatment would require the use of delay differential equations.
Effective reproduction number
In reality, varying proportions of the population are immune to any given disease at any given time. To account for this, the effective reproduction number or
is used.
is the average number of new infections caused by a single infected individual at time t in the partially susceptible population. It can be found by multiplying
by the fraction S of the population that is susceptible. When the fraction of the population that is immune increases (i. e. the susceptible population S decreases) so much that
drops below 1 in a basic SIR simulation, "herd immunity" has been achieved and the number of cases occurring in the population will gradually decrease to zero.
Limitations of R0
Use of in the popular press has led to misunderstandings and distortions of its meaning.
can be calculated from many different mathematical models. Each of these can give a different estimate of
, which needs to be interpreted in the context of that model. Therefore, the contagiousness of different infectious agents cannot be compared without recalculating
with invariant assumptions.
values for past outbreaks might not be valid for current outbreaks of the same disease. Generally speaking,
can be used as a threshold, even if calculated with different methods: if
, the outbreak will die out, and if
, the outbreak will expand. In some cases, for some models, values of
can still lead to self-perpetuating outbreaks. This is particularly problematic if there are intermediate vectors between hosts (as is the case for zoonoses), such as malaria. Therefore, comparisons between values from the "Values of
of well-known infectious diseases" table should be conducted with caution.
Although cannot be modified through vaccination or other changes in population susceptibility, it can vary based on a number of biological, sociobehavioral, and environmental factors. It can also be modified by physical distancing and other public policy or social interventions, although some historical definitions exclude any deliberate intervention in reducing disease transmission, including nonpharmacological interventions. And indeed, whether nonpharmacological interventions are included in
often depends on the paper, disease, and what if any intervention is being studied. This creates some confusion, because
is not a constant; whereas most mathematical parameters with "nought" subscripts are constants.
depends on many factors, many of which need to be estimated. Each of these factors adds to uncertainty in estimates of
. Many of these factors are not important for informing public policy. Therefore, public policy may be better served by metrics similar to
, but which are more straightforward to estimate, such as doubling time or half-life (
).
Methods used to calculate include the survival function, rearranging the largest eigenvalue of the Jacobian matrix, the next-generation method, calculations from the intrinsic growth rate, existence of the endemic equilibrium, the number of susceptibles at the endemic equilibrium, the average age of infection and the final size equation. Few of these methods agree with one another, even when starting with the same system of differential equations. Even fewer actually calculate the average number of secondary infections. Since
is rarely observed in the field and is usually calculated via a mathematical model, this severely limits its usefulness.
Sample values for various infectious diseases
Despite the difficulties in estimating mentioned in the previous section, estimates have been made for a number of genera, and are shown in this table. Each genus may be composed of many species, strains, or variants. Estimations of
for species, strains, and variants are typically less accurate than for genera, and so are provided in separate tables below for diseases of particular interest (influenza and COVID-19).
Disease | Transmission | R0 | HIT |
---|---|---|---|
Measles | Aerosol | 12–18 | 92–94% |
Chickenpox (varicella) | Aerosol | 10–12 | 90–92% |
Mumps | Respiratory droplets | 10–12 | 90–92% |
Rubella | Respiratory droplets | 6–7 | 83–86% |
Polio | Fecal–oral route | 5–7 | 80–86% |
Pertussis | Respiratory droplets | 5.5 | 82% |
Smallpox | Respiratory droplets | 3.5–6.0 | 71–83% |
HIV/AIDS | Body fluids | 2–5 | 50–80% |
COVID-19 (ancestral strain) | Respiratory droplets and aerosol | 2.9 (2.4–3.4) | 65% (58–71%) |
SARS | Respiratory droplets | 2–4 | 50–75% |
Diphtheria | Saliva | 2.6 (1.7–4.3) | 62% (41–77%) |
Common cold (e.g., rhinovirus) | Respiratory droplets | 2–3 | 50–67% |
Mpox | Physical contact, body fluids, respiratory droplets | 2.1 (1.5–2.7) | 53% (31–63%) |
Ebola (2014 outbreak) | Body fluids | 1.8 (1.4–1.8) | 44% (31–44%) |
Influenza (seasonal strains) | Respiratory droplets | 1.3 (1.2–1.4) | 23% (17–29%) |
Andes hantavirus | Respiratory droplets and body fluids | 1.2 (0.8–1.6) | 16% (0–36%) |
Nipah virus | Body fluids | 0.5 | 0% |
MERS | Respiratory droplets | 0.5 (0.3–0.8) | 0% |
Estimates for strains of influenza.
Disease | Transmission | R0 | HIT |
---|---|---|---|
Influenza (1918 pandemic strain) | Respiratory droplets | 2 | 50% |
Influenza (2009 pandemic strain) | Respiratory droplets | 1.6 (1.3–2.0) | 37% (25–51%) |
Influenza (seasonal strains) | Respiratory droplets | 1.3 (1.2–1.4) | 23% (17–29%) |
Estimates for variants of SARS-CoV-2.
Disease | Transmission | R0 | HIT |
---|---|---|---|
COVID-19 (Omicron variant) | Respiratory droplets and aerosol | 9.5 | 89% |
COVID-19 (Delta variant) | Respiratory droplets and aerosol | 5.1 | 80% |
COVID-19 (Alpha variant) | Respiratory droplets and aerosol | 4–5 | 75–80% |
COVID-19 (ancestral strain) | Respiratory droplets and aerosol | 2.9 (2.4–3.4) | 65% (58–71%) |
In popular culture
In the 2011 film Contagion, a fictional medical disaster thriller, a blogger's calculations for are presented to reflect the progression of a fatal viral infection from isolated cases to a pandemic.
See also
-
Compartmental models in epidemiology describe disease dynamics over time in a population of susceptible (S), infectious (I), and recovered (R) people using the SIR model. Note that in the SIR model,
and
are different quantities – the former describes the number of recovered at t = 0 whereas the latter describes the ratio between the frequency of contacts to the frequency of recovery.
- Held L, Hens N, O'Neill PD, Wallinga J (November 7, 2019). Handbook of Infectious Disease Data Analysis. CRC Press. p. 347. ISBN 978-1-351-83932-7. According to Guangdong Provincial Center for Disease Control and Prevention, "The effective reproductive number (R or Re is more commonly used to describe transmissibility, which is defined as the average number of secondary cases generated by per [sic] infectious case." For example, by one preliminary estimate during the ongoing pandemic, the effective reproductive number for SARS-CoV-2 was found to be 2.9, whereas for SARS it was 1.77.
Further reading
- Heesterbeek, J.A.P. (2002). "A brief history of R0 and a recipe for its calculation". Acta Biotheoretica. 50 (3): 189–204. doi:10.1023/a:1016599411804. hdl:1874/383700. PMID 12211331. S2CID 10178944.
- Heffernan, J.M; Smith, R.J; Wahl, L.M (September 22, 2005). "Perspectives on the basic reproductive ratio". Journal of the Royal Society Interface. 2 (4): 281–293. doi:10.1098/rsif.2005.0042. PMC 1578275. PMID 16849186.
-
Jones JH (May 1, 2007). "Notes on
" (PDF). Retrieved November 6, 2018.
- Van Den Driessche, P.; Watmough, James (2008). "Further Notes on the Basic Reproduction Number". Mathematical Epidemiology. Lecture Notes in Mathematics. Vol. 1945. pp. 159–178. doi:10.1007/978-3-540-78911-6_6. ISBN 978-3-540-78910-9.
Concepts in infectious disease
| |||||||||||
---|---|---|---|---|---|---|---|---|---|---|---|
Determinants |
|
||||||||||
Transmission |
|
||||||||||
Prevention and Control measures |
|
||||||||||
Emerging infections | |||||||||||
Other |