Продолжая использовать сайт, вы даете свое согласие на работу с этими файлами.
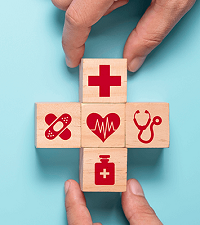
Druggability
Druggability is a term used in drug discovery to describe a biological target (such as a protein) that is known to or is predicted to bind with high affinity to a drug. Furthermore, by definition, the binding of the drug to a druggable target must alter the function of the target with a therapeutic benefit to the patient. The concept of druggability is most often restricted to small molecules (low molecular weight organic substances) but also has been extended to include biologic medical products such as therapeutic monoclonal antibodies.
Drug discovery comprises a number of stages that lead from a biological hypothesis to an approved drug. Target identification is typically the starting point of the modern drug discovery process. Candidate targets may be selected based on a variety of experimental criteria. These criteria may include disease linkage (mutations in the protein are known to cause a disease), mechanistic rationale (for example, the protein is part of a regulatory pathway that is involved in the disease process), or genetic screens in model organisms. Disease relevance alone however is insufficient for a protein to become a drug target. In addition, the target must be druggable.
Prediction of druggability
If a drug has already been identified for a target, that target is by definition druggable. If no known drugs bind to a target, then druggability is implied or predicted using different methods that rely on evolutionary relationships, 3D-structural properties or other descriptors.
Precedence-based
A protein is predicted to be "druggable" if it is a member of a protein family for which other members of the family are known to be targeted by drugs (i.e., "guilt" by association). While this is a useful approximation of druggability, this definition has limitations for two main reasons: (1) it highlights only historically successful proteins, ignoring the possibility of a perfectly druggable, but yet undrugged protein family; and (2) assumes that all protein family members are equally druggable.
Structure-based
This relies on the availability of experimentally determined 3D structures or high quality homology models. A number of methods exist for this assessment of druggability but all of them consist of three main components:
- Identifying cavities or pockets on the structure
- Calculating physicochemical and geometric properties of the pocket
- Assessing how these properties fit a training set of known druggable targets, typically using machine learning algorithms
Early work on introducing some of the parameters of structure-based druggability came from Abagyan and coworkers and then Fesik and coworkers, the latter by assessing the correlation of certain physicochemical parameters with hits from an NMR-based fragment screen. There has since been a number of publications reporting related methodologies.
There are several commercial tools and databases for structure-based druggability assessment. A publicly available database of pre-calculated druggability assessments for all structural domains within the Protein Data Bank (PDB) is provided through the ChEMBL's DrugEBIlity portal.
Structure-based druggability is usually used to identify suitable binding pocket for a small molecule; however, some studies have assessed 3D structures for the availability of grooves suitable for binding helical mimetics. This is an increasingly popular approach in addressing the druggability of protein-protein interactions.
Predictions based on other properties
As well as using 3D structure and family precedence, it is possible to estimate druggability using other properties of a protein such as features derived from the amino-acid sequence (feature-based druggability) which is applicable to assessing small-molecule based druggability or biotherapeutic-based druggability or the properties of ligands or compounds known to bind the protein (Ligand-based druggability).
The importance of training sets
All methods for assessing druggability are highly dependent on the training sets used to develop them. This highlights an important caveat in all the methods discussed above: which is that they have learned from the successes so far. The training sets are typically either databases of curated drug targets; screened targets databases (ChEMBL, BindingDB, PubChem etc.); or on manually compiled sets of 3D structure known by the developers to be druggable. As training sets improve and expand, the boundaries of druggability may also be expanded.
Undruggable targets
Only 2% of human proteins interact with currently approved drugs. Furthermore, it is estimated that only 10-15% of human proteins are disease modifying while only 10-15% are druggable (there is no correlation between the two), meaning that only between 1-2.25% of disease modifying proteins are likely to be druggable. Hence it appears that the number of new undiscovered drug targets is very limited.
A potentially much larger percentage of proteins could be made druggable if protein–protein interactions could be disrupted by small molecules. However the majority of these interactions occur between relatively flat surfaces of the interacting protein partners and it is very difficult for small molecules to bind with high affinity to these surfaces. Hence these types of binding sites on proteins are generally thought to be undruggable but there has been some progress (by 2009) targeting these sites.
Chemoproteomics techniques have recently expanded the scope of what is deemed a druggable target through the identification of covalently modifiable sites across the proteome.
Further reading
- Griffith M, Griffith OL, Coffman AC, Weible JV, McMichael JF, Spies NC, Koval J, Das I, Callaway MB, Eldred JM, Miller CA, Subramanian J, Govindan R, Kumar RD, Bose R, Ding L, Walker JR, Larson DE, Dooling DJ, Smith SM, Ley TJ, Mardis ER, Wilson RK (2013). "DGIdb: mining the druggable genome". Nature Methods. 10 (12): 1209–10. doi:10.1038/nmeth.2689. PMC 3851581. PMID 24122041.
- Wagner AH, Coffman AC, Ainscough BJ, Spies NC, Skidmore ZL, Campbell KM, Krysiak K, Pan D, McMichael JF, Eldred JM, Walker JR, Wilson RK, Mardis ER, Griffith M, Griffith OL (2016). "DGIdb 2.0: mining clinically relevant drug-gene interactions". Nucleic Acids Research. 44 (D1): D1036–44. doi:10.1093/nar/gkv1165. PMC 4702839. PMID 26531824.
External links
- "DrugEBIlity". ChEMBL.
- "The Drug Gene Interaction Database (DGIdb)". Washington University School of Medicine.
- "TDR Targets Database". The TDR Drug Targets Network.